LLM for Recommender System
Wenyue Hua
May 10, 2023
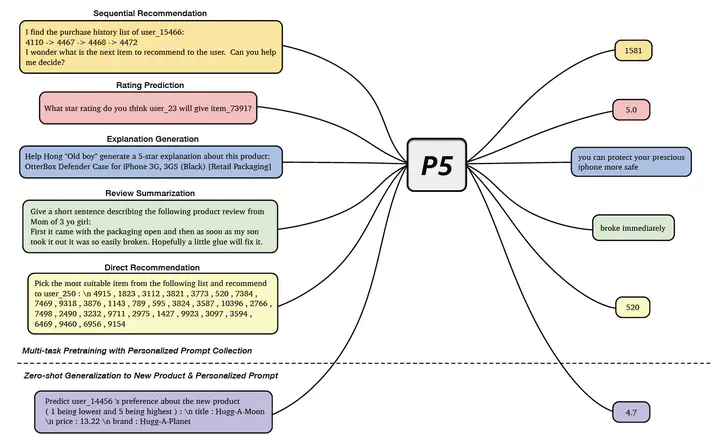
Abstract
The integration of foundation models like Large Language Models (LLMs) into recommender systems (RS) marks a significant advancement in the field. Adapting LLMs to recommender systems that manage billions of users and items presents a complex yet crucial challenge. This exploration delves into the benefits and potential issues of utilizing LLMs within recommender systems, contributing to advancements in this area.
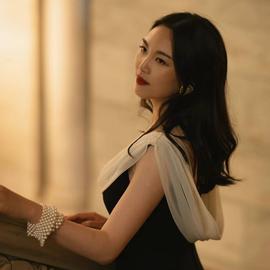
Wenyue Hua
Postdoctoral Researcher
Ph.D. in artificial intelligence, specifically focused on large language models.
Publications
This paper presents OpenP5, an open-source library for benchmarking foundation models for recommendation under the Pre-train, Personalized Prompt and Predict Paradigm (P5). We consider the implementation of P5 on three dimensions – 1) downstream task, 2) recommendation dataset, and 3) item indexing method. For 1), we provide implementation over two downstream tasks – sequential recommendation and straightforward recommendation. For 2), we surveyed frequently used datasets in recommender system research in recent years and provide implementation on ten datasets. In particular, we provide both single-dataset implementation and the corresponding checkpoints (P5) and another Super P5 (SP5) implementation that is pre-trained on all of the datasets, which supports recommendation across various domains with one model. For 3), we provide implementation of three item indexing methods to create item IDs – random indexing, sequential indexing, and collaborative indexing. We also provide comprehensive evaluation results of the library over the two downstream tasks, the ten datasets, and the three item indexing methods to facilitate reproducibility and future research. We open-source the code and the pre-trained checkpoints of the OpenP5 library at this url.
Shuyuan Xu,
Wenyue Hua,
Yongfeng Zhang
Recommendation foundation model utilizes large language models (LLM) for recommendation by converting recommendation tasks into natural language tasks. It enables generative recommendation which directly generates the item(s) to recommend rather than calculating a ranking score for each and every candidate item as in traditional recommendation models, simplifying the recommendation pipeline from multi-stage filtering to single-stage filtering. To avoid generating excessively long text and hallucinated recommendations when deciding which item(s) to recommend, creating LLM-compatible item IDs to uniquely identify each item is essential for recommendation foundation models. In this study, we systematically examine the item ID creation and indexing problem for recommendation foundation models, using P5 as an example of the backbone LLM. To emphasize the importance of item indexing, we first discuss the issues of several trivial item indexing methods, such as random indexing, title indexing, and independent indexing. We then propose four simple yet effective solutions, including sequential indexing, collaborative indexing, semantic (content-based) indexing, and hybrid indexing. Our study highlights the significant influence of item indexing methods on the performance of LLM-based recommendation, and our results on real-world datasets validate the effectiveness of our proposed solutions. The research also demonstrates how recent advances on language modeling and traditional IR principles such as indexing can help each other for better learning and inference. Source code and data are available at this url.
Wenyue Hua,
Shuyuan Xu,
Yingqiang Ge,
Yongfeng Zhang